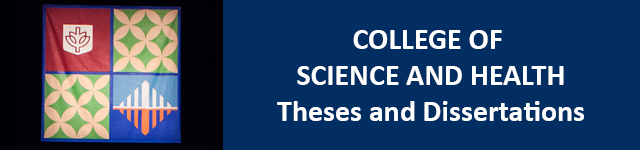
Date of Award
Spring 6-11-2021
Degree Type
Thesis
Degree Name
Master of Science (MS)
Department
Physics
First Advisor
Eric Landahl, PhD
Second Advisor
Christopher Goedde, PhD
Third Advisor
Gabriela Gonzalez Aviles, PhD
Abstract
In soft-matter physics, Dynamic Light Scattering (DLS) stands out as the non-invasive technique designed to measure the size and distribution of particles using speckle correlation. Traditional DLS analysis performs a one-to-one mapping of the size with diffusion which is computationally costly, in addition to lacks in generalisation to seek deeper relationships amongst speckle patterns. A recent advancement in double-pulse speckle imaging allows for the temporal-correlation problem presented by DLS to be transformed into an image classification problem. In this prototype study, I probe the feasibility and efficacy of DLS data analysis of speckle correlation using Deep Learning (DL). To reduce the loss of generality and retain the integrity of the speckles, the study implements an image-focused DL algorithm. A Convolutional Neural Network (CNN or ConvNet) is used for feature extraction of pixelated images that are time-series in nature. Each scan consists of 500 frames (images) taken with double-pulses of 100 nanoseconds duration at 17 time delays. The CNN model was trained with roughly 1000 observations per sample with labelled target to supervise the training. The model picked up patterns within image matrices producing best performance accuracy of 83, average accuracy hovered at 58 percent. Treating speckle images to artificial intelligence to classify to the impurity type opens a new avenue to perform analysis in DLS. This prototype study shows that CNN could be an alternative candidate to traditional speckle image analysis.
Recommended Citation
Basnet, Sanskar, "SPECKLE CORRELATION USING A DEEP LEARNING ALGORITHM ON NEWTONIAN FLUIDS" (2021). College of Science and Health Theses and Dissertations. 383.
https://via.library.depaul.edu/csh_etd/383
SLP Collection
no