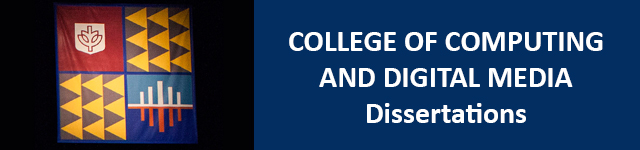
Date of Award
Spring 5-27-2022
Degree Type
Thesis
Degree Name
Master of Science (MS)
School
School of Computing
First Advisor
Roselyne Tchoua, PhD
Second Advisor
Jacob Furst, PhD
Third Advisor
Daniela Raicu, PhD
Fourth Advisor
Peter Hastings, PhD
Abstract
Despite the exponential growth in scientific textual content, research publications are still the primary means for disseminating vital discoveries to experts within their respective fields. These texts are predominantly written for human consumption resulting in two primary challenges; experts cannot efficiently remain well-informed to leverage the latest discoveries, and applications that rely on valuable insights buried in these texts cannot effectively build upon published results. As a result, scientific progress stalls. Automatic Text Summarization (ATS) and Information Extraction (IE) are two essential fields that address this problem. While the two research topics are often studied independently, this work proposes to look at ATS in the context of IE, specifically in relation to Scientific IE. However, Scientific IE faces several challenges, chiefly, the scarcity of relevant entities and insufficient training data. In this paper, we focus on extractive ATS, which identifies the most valuable sentences from textual content for the purpose of ultimately extracting scientific relations. We account for the associated challenges by means of an ensemble method through the integration of three weakly supervised learning models, one for each entity of the target relation. It is important to note that while the relation is well defined, we do not require previously annotated data for the entities composing the relation. Our central objective is to generate balanced training data, which many advanced natural language processing models require. We apply our idea in the domain of materials science, extracting the polymer-glass transition temperature relation and achieve 94.7% recall (i.e., sentences that contain relations annotated by humans), while reducing the text by 99.3% of the original document.
Recommended Citation
Keller, Abigail, "Text summarization towards scientific information extraction" (2022). College of Computing and Digital Media Dissertations. 40.
https://via.library.depaul.edu/cdm_etd/40