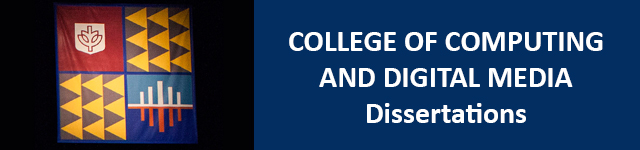
Date of Award
Spring 5-12-2021
Degree Type
Dissertation
Degree Name
Doctor of Philosophy (PhD)
School
School of Computing
First Advisor
Jacob Furst, PhD
Second Advisor
Brian Keegan, PhD
Third Advisor
Sheena Erete, PhD
Fourth Advisor
John Rogers, PhD
Abstract
This research began as an investigation of the #metoo movement, with the initial impetus to illuminate the voices located on the margins, those who often go unheard or are never recognized. This work aimed to understand the intersectional aspects of how these hashtag variations of the hashtag #metoo (i.e. #metoomosque, #churchtoo, #metoodisable, #metooqueer, #metoochina, etc) reveal the inequities of the #metoo movement on Twitter. The proliferation of these hashtag variations has often been ignored by scholars, and therefore absorbed into the larger #metoo movement conversation on Twitter. Therefore, the term `hashtag derivative' was created to describe the variation on the theme of its original hashtag, strongly reflecting its composition.
Moreover, a critical theory such as Intersectionality is well-equipped to explore how overlapping identities encounter structure social reality relationship to power. Amid a pandemic and racial unrest, the true capabilities of Intersectionality to describe inequities and injustices beyond the singular social position of race and gender are not widely understood. Data science, is not absolved of its role in inequities and injustices merely by dint of being a quantitative field that claims to ``objectivity''. Social scientists have illuminated the racism, sexism, ableism, transphobia, homophobia, prejudice, bigotry, and bias embedded in data science's technology, tools, and algorithms. This has, direct and indirectly, grave consequences on an entire community as a whole as well as marginalized communities.
The application of Intersectionality into a quantitative field can provide researchers a formal structure to be more conscientious about how to critique, develop, and design their data science processes, while also reckoning with their own positioning in relationship to the data. In this way, Intersectionality is inclusive in terms of data equity yet adds an additional layer of accountability to the researcher. This research leads to the three critical contributions of this work: (1) creating a more concise terminology to describe the phenomenon of hashtag variation, known as hashtag derivatives, (2) defining the historical context of Intersectionality and building a formal case for this to be properly contextualized in the Computer Science field (in particular Data Science), and (3) developing the Quantitative Intersectional Data (QUINTA) Framework which data scientists and scholars can use to be more equitable, inclusive and accountable for their role in the data science process.
Recommended Citation
Boyd, Alicia E., "Quantitative intersectional data (QUINTA): a #metoo case study" (2021). College of Computing and Digital Media Dissertations. 36.
https://via.library.depaul.edu/cdm_etd/36