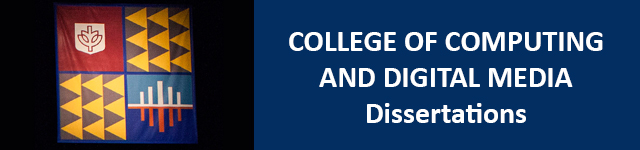
Date of Award
Winter 11-15-2019
Degree Type
Thesis
Degree Name
Master of Science (MS)
School
School of Computing
First Advisor
Daniela Raicu, PhD
Second Advisor
Jacob Furst, PhD
Third Advisor
Enid Montague, PhD
Abstract
The extent of electronic health record systems usage in clinical settings has affected the dynamic between clinicians and patients and has thus been connected to physician morale and the quality of care patients receive. Recent research has also uncovered a correlation between physician burnout and negative physician attitudes electronic health record systems. In order to begin exploring the nature of the relationship between electronic health record usage, physician burnout, and patient care, it is necessary to first analyze patient-provider interactions within the context of verbal features such as turn-taking and non-verbal features such as eye-contact. While previous works have sought to annotate non-verbal and verbal features via manual coding techniques and then analyze their impacts, we seek to automate the process of annotation in order to create a more robust system of analysis in less time-consuming fashion.
This research thesis focuses upon physician gaze and speaking annotations, as these are non-verbal and verbal components of the interaction which can be connected to eye-contact and turn-taking, respectively, which are themselves features that have linked in certain research to patient outcomes. Previously published work from within this project has demonstrated the viability of extracting image features in the form of YOLO-based person positioning coordinates and optical flow summary statistics to inform the learning of physician gaze for two physicians and six patients with over 80% minimum accuracy. The work described in this thesis expands upon the previous findings by increasing the number of patients and physicians in the realm of analysis; by diversifying the classifiers to be more robust to new data; and by incorporating automatically extracted audio information in the form of mel frequency cepstral coefficients and its derivatives, as well as an additional optical flow summary statistic, in order to make predictions regarding physician gaze and speaking annotations on a frame by frame basis. We thus illustrate a process of developing and implementing an automated system for multiple video labeling of physician-patient interactions. In so doing, we demonstrate that a combination of audio and visual features can be combined to inform the predictions of physician gaze and speaking annotations in both testing and sequential validation data. While our approach focuses upon learning physician gaze and speaking annotations, the methodologies introduced can be extended to capture other aspects of the interaction as well as connect these interactions to patient ratings of clinical interactions, physician usage of electronic health record systems, and measures of physician burnout. Ultimately, the approaches presented in this paper can aid the creation of an interactive system providing instantaneous feedback to providers during clinician visits, which will be created with the intention of improving clinical care within the context of electronic health care so as to enhance care, improve patient outcomes, and reduce instances of physician burnout.
Recommended Citation
Gutstein, Daniel Baruch, "Information extraction from primary care visits to support patient-provider interactions" (2019). College of Computing and Digital Media Dissertations. 26.
https://via.library.depaul.edu/cdm_etd/26