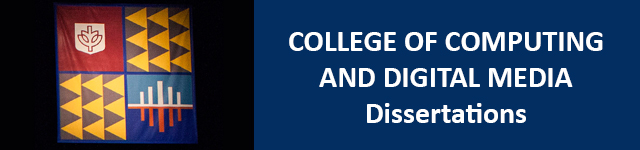
Date of Award
Spring 6-10-2012
Degree Type
Dissertation
Degree Name
Doctor of Philosophy (PhD)
School
School of Computing
First Advisor
Dr. Susy Chan
Second Advisor
Dr. Xiaowen Fang
Abstract
Many disciplines in everyday life depend on improved performance in conditional probability problems. Most adults struggle with conditional probability problems and several prior studies have shown participant accuracy is less than 50%. This study examined user performance when aided with computer-generated Venn and Euler type diagrams in a non-learning context. Despite the prevalence of research into diagrams and extensive research into conditional probability problem solving, this study is one of the only studies to apply theories of working memory to predict user performance in conditional probability problems with diagrams. Following relational complexity theory, this study manipulated problem complexity in computer generated diagrams and text-only displays to improve user performance and perceptions of satisfaction. Partially consistent with the study hypotheses, complex visuals outperformed complex text-only displays and simple text-only displays outperformed complex text-only displays. However, a significant interaction between users’ spatial ability and the use of diagram displays led to a degradation of low-spatial user performance in the diagram displays when compared to high spatial users. Participants with less spatial ability were significantly impaired in their ability to solve conditional probability problems when aided by a diagram.
Recommended Citation
Kellen, Vincent J., "The Effects of Diagrams and Relational Complexity on User Performance in Conditional Probability Problems in a Non-Learning Context" (2012). College of Computing and Digital Media Dissertations. 2.
https://via.library.depaul.edu/cdm_etd/2
Included in
Cognitive Psychology Commons, Graphic Communications Commons, Graphics and Human Computer Interfaces Commons, Health Communication Commons