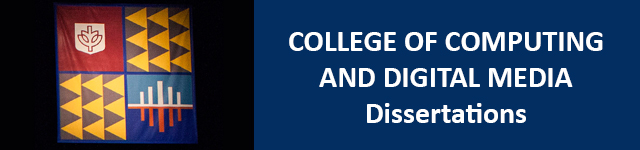
Date of Award
Fall 11-22-2021
Degree Type
Thesis
Degree Name
Master of Science (MS)
School
School of Computing
First Advisor
Ilyas Ustun, PhD
Second Advisor
Jacob Furst, PhD
Third Advisor
Rafaella Settimi-Woods, PhD
Abstract
Computational progressive failure analysis (PFA) is vital for the design, verification, and validation of carbon fiber reinforced polymer (CFRP) composites. However, the computational cost of PFA is usually high due to the complexity of the model. The damage initiation criterion is one of the essential components of a PFA code to determine the transition of a material’s state from pristine or microscopically damaged to macroscopically damaged. In this thesis, data-driven models are developed to determine the matrix damage initiation based on the Mohr-Coulomb model and Hashin model. For 2D plane stress states, the computational cost for determining damage initiation can be dramatically reduced by implementing a Binary Search (BS) algorithm and predictive machine learning models. We have demonstrated the usage of BS and the training and evaluation of machine learning models as alternative methods to determine macroscale matrix damage initiation. With the data-driven methods, over 99% of the computational time has been saved, while the predictive accuracy stays 99.9% from the traditional approach. For a 3D stress state, 87% of the computational time is saved with the predictive accuracy of 94.7%.
Recommended Citation
Post, Alexander Richard, "Data-driven damage initiation criteria for carbon fiber reinforced polymer composites" (2021). College of Computing and Digital Media Dissertations. 44.
https://via.library.depaul.edu/cdm_etd/44